Collaborators: Dr. Dan Gillis, Dr. Theresa Bernardo, Matthew Kreitzer, Rashi Mathur, Xavier Ifill, Luc Dube
Job Title: Undergraduate Data Scientist
University: University of Guelph
Note this is a current project. Progress on it is still being made.
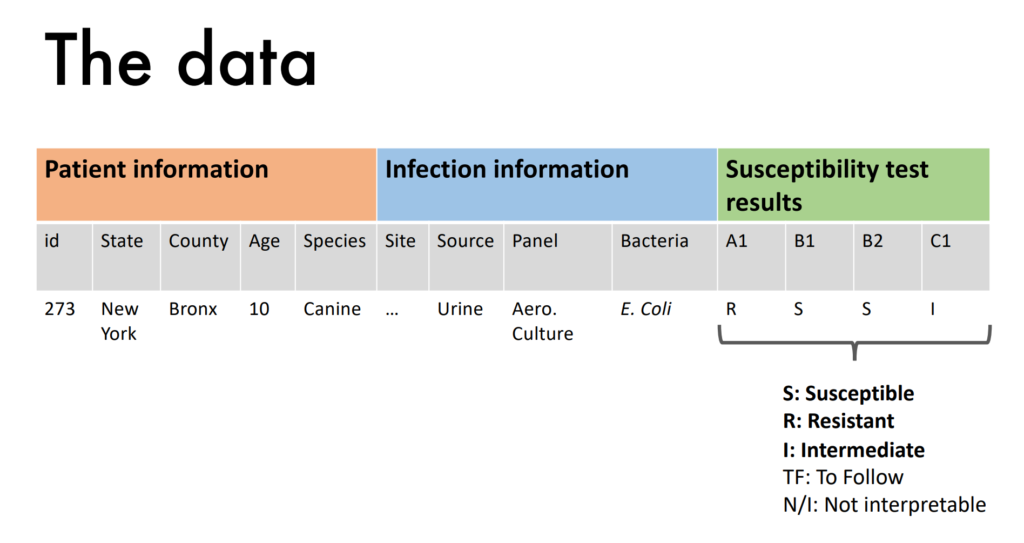
Problem / Description
- The widespread and often unnecessary use of antibiotics in humans, livestock, and agriculture accelerates the evolution of bacteria, leading to the development of resistant strains, making antibacterial resistance progressively worse.
- Specifically, the overuse of antibacterials on companion animals is under researched.
- Research team of Dr. Theresa Bernardo and Matthew Kreitzer, is working with the class of CIS*4020 to create a dashboard prototype that vets can use to predict which drugs are resisted by which bacteria.
- We are given access to a private dataset of patient information, and infection information as features, and are tasked on predicting susceptibility test results.
Method
- We designed an experiment to compare the efficacy of neural networks vs. k-clustering techniques on predicting antibacterial resistance.
- We find large gains in both of the models, by adding interdependent meta information from other entries in the dataset. (e.g. How do patients near you effect the diseases you can get? How does diseases change over time? How do repeat patients differ from one-time patients?)
- We will present our findings in a dashboard, and a presentation to the research team.
Results
- Results are not yet public. Will be updated